
Algorithmic Play
Education and Digital Culture 2020
University of Edinburgh-Digital Education
s1891551
THE
NETFLIX™ ALGORITHM
How has the algorithm affected the options you are given or what you can see?
I have been using Netflix™ for a while so I managed to collect data for the month of February through the email suggestions the program sends to my email account. The Netflix™ algorithm seems to post most suggestions on Mondays and Fridays. Netflix's algorithm basis its recommendations on a number of factors.
Data generated by the watched history is one of the main determinants. Rating of titles is another but since I never rate titles, this did not generate much data on my part. Recommendations are not based on my humble preferences but are generated together with other viewers; likes. Movie genre, categories, actors, actors and release years are also important variables. Netflix provides a Percentage Score that calculates the probability that a user will like (or not) a particular title.
What is amazing is that Netflix™ also takes into account the time of day I watch, the devices from which I watch and how long I watch.
Netflix™ also uses a ranking system like Amazon. It ranks movies within a row and between rows.
The top rows are the ones most strongly recommended while the most strongly recommended titles go to the left.
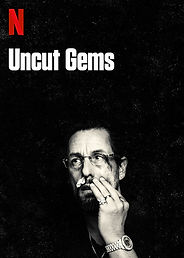
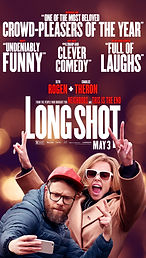

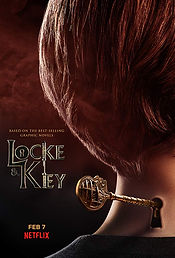
How have your actions changed what the algorithm has done?
Monday 3th Feb
Friday 7th Feb
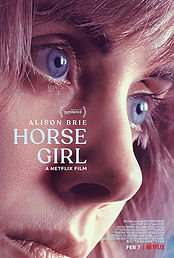
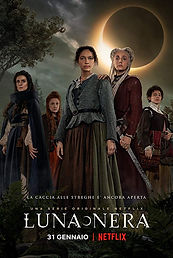

Monday 17th Feb
Analysing my choice of movies.
I like thrillers, mystery and horror movies and the occasional funny movie. While I enjoy watching something most evenings, I usually fall asleep after a short time.
It seems that Netflix's™ algorithm has detected that Mondays and Fridays are the days I watch most.
Most of the recommendations are also movies that fall into the genres mentioned above.

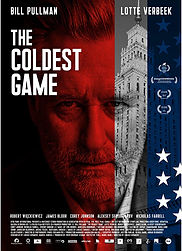

The BFG entry this week is how Netflix™ seems to have detected that my daughters used my account rather than theirs. It is the only suggestion related to kids this week.
Out of all the recommendations received I only watched The Coldest Game which I recommend. Once again another recommendation is for an action movie called the Last Witchunter.
Friday 21st Feb
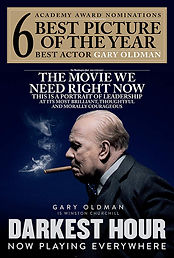

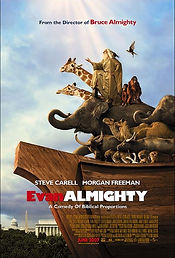
While I do enjoy the occasional nature documentary, I am not a great fan, yet it still recommended one. I tried to remember if there was anything connected to Romania rather than nature documentaries which might have triggered the recommendation.
It seems that Netflix™ caught my daughters on my account yet again as can be evidenced by the movie suggestion Evan Almighty which they have watched over and over.
Monday 24th Feb
How have other people been involved in shaping results?
The algorithm behind Netflix's™ choices and recommendations is similar to other algorithms used by content management sites in that it takes into consideration personal choices and compares them to choices made by others. The algorithm is powerful as it is not static but morphs and develops according to user watching trends which are used, in turn, to recommend content that it assumes particular individuals will enjoy. The algorithm is also supported by humans who place content into groups and tag it in order to determine specific genres and micro genres in order to provide more specific recommendations through the Netflix platform of rows and columns. This means that Netflix users are presented with very exact recommendations at a glance.
Netflix™ Privacy Policy collects different types of information from users, including activity, contact messages, device IDs, device characteristics and cookies. Netflix™ can also collect information relating to your ISP, mobile phone carrier and even bank. It uses this data to determine where you are, prevent illegal activity and store your preferences. Giving access to others will make your watch history available to them. In other words, Netflix™ encourages multiple accounts to be used within one household rather than shared outside the home.
Netflix™ acts like a personal viewing portfolio. It needs the types of data mentioned above to provide personalised results. Some of the data collected, such as location information may seem too much but this allows Netflix™ to provide content based on location and country. This is all made clear in the privacy policy. Furthermore, some devices may receive 'Push notifications' for advertising or promotions. Netflix™ uses a system of privacy-protective identifiers to pass on user information to third parties without revealing private data. This is done so Netflix™ can send promotions.
While Netflix™ makes its data usage policy clear, there is a considerable amount of information that is collected from individuals. It is the price that is paid for a tailor-made service. Would Netflix™ users agree to a less tailor-made solution if given the choice to reveal less data about themselves? Probably not. Getting personalised recommendations saves on time searching and keeps users updated. It also shows what others are watching and what the public likes, thereby encouraging individuals to be 'like others' and follow the most popular series or the latest films.
What are the ethical issues at stake with your chosen algorithm? Is there data here that should be private?
What are the implications for digital education implied by your chosen algorithm?
How does Netflix™ compare to the use of datafication in education?
Knox (2015) questions the objectivity of these types of algorithms which seem to create their own 'worlds' and therefore are a symbol of power. Similarly, Kitchin (2017) agrees to the power of algorithms, 'wherein algorithms will play an ever increasing role in the exercise of power, a means through which to automate the disciplining and controlling of societies and to increase the efficiency of capital accumulation.' This can be better understood if one observes the many social, political and economic influences the learning experience has to pass through in order to be digitized and served to learners.
On the other hand, this power enables a vision of a modern form of education, such as the one envisaged by Mayer -Schonberger and Cukier (cited in Williamson, 2017) of 'individualization and personalization of the educational experience through adaptive learning systems that enable materials to be tailored to each student's individual needs through automated real-time analysis'. This could be a system that, similarly to the algorithm employed by Netflix™, morphs with every meed through machine learning and reinforcement learning, a 'data-driven education system' (Williamson, 2017).
Algorithms like the one behind Netflix™ gives the impression that the product is there for the user, satisfying his/her every entertainment need. The illusion that such algorithms give can alienate users from the very simple fact that while users are getting recommendations, they are also 'nudged' or gently convinced into watching a particular kind of content. A case in point is the Percentage Score system which assumes that a user will like a movie based on data gathered from a number of sources. Movies gain popularity by being 'pushed' in front of the user as recommendations. Basically, rather than having the user go to the product, it is bringing the product to the user and so makes it more attractive. Powerful platform like Netflix™ and similar ones in the educational systems of tomorrow create 'sociotechinical imaginaries' (Williamson, 2017) that are 'produced by particular social groups within specific social contexts...' and so will always wonder as to their objectivity.
Let us imagine for a second that instead of entertainment, this was an e-learning platform or a MOOC. It would generate learning experiences based on learning history and popular learning courses. It would put recommended courses in the line of sight to entice learners into clicking on them. It would encourage one course after another, encourage payment for certificates or for the continuation of a course. In other words, such algorithms have a 'disruptive and transformative effect, reconfiguring how systems operate, enacting new forms of electronic governance and enabling new forms of capital accumulation'. (Kitchin, 2017). Couple this with the possibility of having advertising (even if filtered) placed here and there and a the learning platform becomes an opportunity for subtle marketing.